Elasticsearch 9.0 & 8.18: Cooked for developers, with another helping of blazing-fast BBQ — 5x faster than OpenSearch
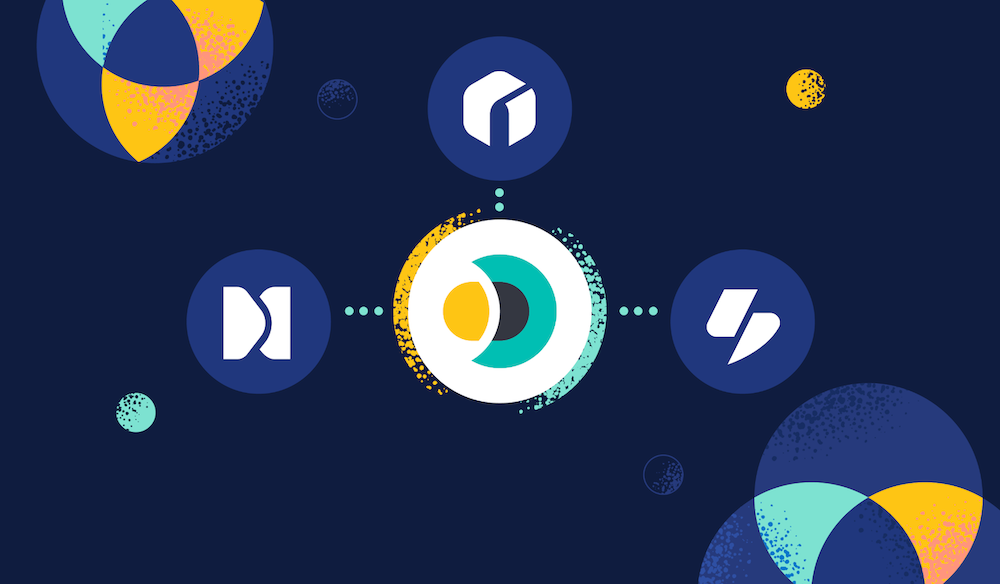
We are proud to be releasing version Elasticsearch 9.0 and 8.18 to Elastic Cloud and self-managed users. The capabilities in these releases have already been available to our Elastic Cloud Serverless users, who have had access to generally available fully managed Elasticsearch on AWS, Azure, and GCP.
Serving Better Binary Quantization (BBQ) even faster — 5x faster than OpenSearch
BBQ (Better Binary Quantization), first introduced in 8.16 as a technical preview, is now generally available, offering a high-performance alternative to traditional quantization techniques like Product Quantization (PQ).
Our customers, like Roboflow, store and update increasingly larger amounts (i.e., billions) of vector data, and in the past would have had to consider using something like PQ to maintain their relevance and performance but more effectively utilize their existing hardware. Now, they have access to BBQ.
BBQ now has an updated algorithm delivering up to 20% higher recall and 8x–30x faster throughput with SIMD for efficient, accurate search. Elastic is the first vector database vendor to implement this approach, enabling real-world search workloads to achieve faster results while reducing computing resources.
As part of our mission to make Apache Lucene the best vector database, and as champions of bringing these innovations to the community, we have recently merged these capabilities to Lucene.
Compared to OpenSearch FAISS, Elasticsearch BBQ delivers up to 5x faster queries and 3.9x higher throughput across all recall levels, all while maintaining the same accuracy. Designed for speed and efficiency, BBQ significantly lowers latency, making it ideal for large-scale production workloads.
Quantized vector rescoring now features a simplified API, further improving the developer experience. BBQ performs a full index scan using a small predictor vector, oversamples results, and then reranks them using the larger vector. With the new API, simply define the oversampling rate, and let Elasticsearch handle the reranking seamlessly.
With this release, multi-stage interaction models such as ColPali and ColBERT are also now supported with MaxSim!
Out-of-the-box semantic search and semantic reranking
With this release, builders have access out of the box to ELSER, our sparse vector model, and e5, as an optimized multilingual dense vector model for semantic search. Semantic reranking is available also with our Elastic Rerank model, which can be of unique benefit for those wanting to uplevel their relevance without changing the shape of their stored data.
Bringing your own choice of models to add to what you already have from Elastic shouldn’t be a struggle. With our open inference API, it’s easy to use the newly added integration to use the newly contributed JinaAI embeddings and reranking capabilities or Watsonx.ai’s reranking for semantic reranking.
As an example, semantic search starts with a single mapping semantic_text:
PUT my-data
{
"mappings": {
"properties": {
"my_semantic_field": {
"type": "semantic_text" }}}}
By not specifying an inference endpoint, you leverage our default semantic model: ELSER.
If you want to use Jina AI’s latest embedding model jina-embeddings-v3, simply specify the inference endpoint to be used with semantic_text instead. For your convenience, if you’d like to try this yourself, reference this Jupyter notebook to try it out today!
PUT my-data-with-jina
{ "mappings": {
"properties": {
"my_semantic_field": {
"type": "semantic_text",
"inference_id": "my-jinaai-endpoint" }}}}
Now, let’s do your first natural language, human readable semantic search.
POST my-data/_search
{ "query": {
"match": {
"my_semantic_field": "Which vector database was the first in the industry to introduce BBQ and contribute it to the open source community?"
}
},
"highlight": {
"fields": {
"my_semantic_field": {
"number_of_fragments": 2,
"order": "score" }}}}
Or, if you like ES|QL instead, try:
POST _query?format=txt
{
"query": """
FROM my-data
| WHERE my_semantic_field:"Which vector database has BBQ?"
| KEEP my_semantic_field
"""
}
The answer to these queries is of course: Elasticsearch. The choice of whether you use our default model or your favorite is only one inference endpoint definition away.
Wait, there’s more!
In this release, fans of hybrid search will be pleased to know that retrievers — the developer abstraction that we added in previous releases to the query DSL for better composability and ease of use — now have the ability to easily incorporate linear and generic rescoring in retrievers alongside Reciprocal Rank Fusion (RRF), a great default technique for normalizing scores across combinations of different search types.
We continue to add exciting new commands to the Elasticsearch Query Language (ES|QL), but there’s one new command that was not available in the query DSL. Let’s JOIN hands and welcome a new way to query across data with the power of Elasticsearch:
// join employees with their department name
FROM employees
| LOOKUP JOIN departments ON dep_id
| KEEP last_name, first_name, dep_name
Read more about it in the ES|QL JOIN blog!
Try it out
Read about these capabilities and more in the release notes.
Existing Elastic Cloud customers can access many of these features directly from the Elastic Cloud console. Not taking advantage of Elastic on cloud? Start a free trial.
Want to get started fast on your laptop? Run curl -fsSL https://elastic.co/start-local | sh and get going in minutes.
You can also download the Elastic Stack and our cloud orchestration products, Elastic Cloud Enterprise and Elastic Cloud for Kubernetes, for a self-managed experience.
The release and timing of any features or functionality described in this post remain at Elastic's sole discretion. Any features or functionality not currently available may not be delivered on time or at all.
In this blog post, we may have used or referred to third party generative AI tools, which are owned and operated by their respective owners. Elastic does not have any control over the third party tools and we have no responsibility or liability for their content, operation or use, nor for any loss or damage that may arise from your use of such tools. Please exercise caution when using AI tools with personal, sensitive or confidential information. Any data you submit may be used for AI training or other purposes. There is no guarantee that information you provide will be kept secure or confidential. You should familiarize yourself with the privacy practices and terms of use of any generative AI tools prior to use.
Elastic, Elasticsearch, ESRE, Elasticsearch Relevance Engine and associated marks are trademarks, logos or registered trademarks of Elasticsearch N.V. in the United States and other countries. All other company and product names are trademarks, logos or registered trademarks of their respective owners.