Understanding observability metrics: Types, golden signals, and best practices
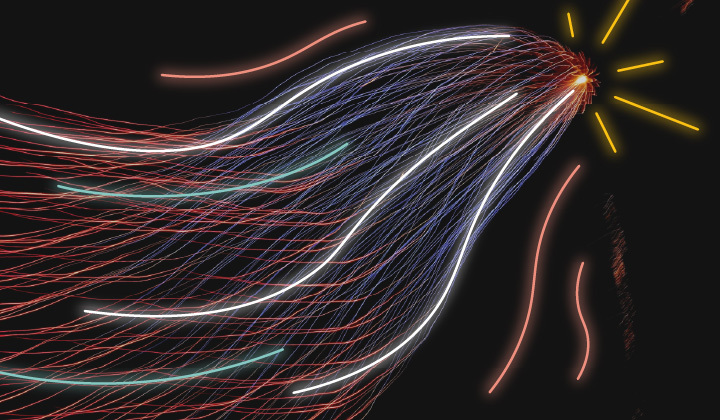
Observability metrics provide insights into the performance, behavior, and health of applications, systems, and infrastructure — enabling observability practices, which is how a system’s internal state is understood by examining its data. As organizations continue to collect more and more data, observability metrics are a key telemetry signal for observability.
In modern application development, observability refers to collecting and analyzing telemetry data — logs, metrics, and traces — from a variety of sources for detailed insight into the behavior of applications running in your environments. Observability metrics are the telemetry signals that help organizations make sense of their operations and create proactive monitoring processes.
By leveraging observability metrics, organizations can obtain a comprehensive view of the performance of their technology stack, improving issue diagnostics and resolution times. When used effectively, observability metrics can provide valuable business insights that drive growth and allow organizations to focus on innovation.
3 pillars of observability
The foundation of observability is often described in terms of three pillars: metrics, logs, and traces. Together, they provide essential visibility into system performance and behavior. As technology continues to advance and observability needs increase, a fourth pillar is emerging: profiles.
Metrics
Metrics are raw numerical data points collected from hardware, software, and websites. In measuring known knowns, metrics are used for monitoring resource usage, performance, and user behavior. In other words, metrics tell monitoring and observability teams what is happening in their systems.
Core types of observability metrics
Observability is a practice that gives organizations a 360-degree view of their environments and operations. To do so, observability relies on these core types of metrics:
Application metrics: Application metrics are the telemetry data generated by and related to applications within a technology stack. Some examples of commonly used metrics include response times, throughput, request rates, and error counts. These metrics allow engineers to monitor application performance and availability. Application metrics are also used in application performance monitoring (APM).
System metrics: System metrics, also referred to as infrastructure metrics, reflect the health of hardware and operating systems including key components like Kubernetes. Examples include CPU utilization, disk I/O, network throughput, memory usage, instance uptime, container resource utilization, and service availability. These metrics provide insights into the performance of cloud resources, virtual machines, containers, and other underlying components.
Business metrics: Business metrics tie technical and operational performance to business outcomes. For example, metrics like conversion rates, average transaction value, and user retention help correlate system performance with organizational objectives.
An effective observability solution ensures reliability, effective resource allocation, compliance, and security. It also helps plan capacity, optimize performance, improve user experiences, and control costs. Core metrics enable effective observability, and ultimately, data-driven decision-making that translates to better business outcomes. These metrics are typically aggregated and visualized in dashboards for real-time performance monitoring.
Logs
Logs are timestamped entries of specific events generated by systems, applications, networks, and infrastructure. They provide event details and context, allowing engineers to understand why issues occur.
Network devices, applications, operating systems, applications, IoT devices, and third-party applications emit different types of logs, including (but not limited to):
System logs: These include events like connection attempts, errors, and configuration changes.
Application logs: They record software changes, CRUD operations, application authentication, and other events to help diagnose issues.
Network logs: Network logs record data from events that take place on a network or device, including network traffic, security events, and user activity.
Logs are recorded in structured and unstructured formats, which represents a storage challenge. They can also be hard to categorize since log data is often siloed in a variety of systems and not automatically correlated.
Traces
Traces are telemetry signals that let engineers see applications and services from a user-session perspective. Distributed tracing collects traces of requests that make their way through a distributed architecture.
Traces allow engineers to monitor and debug applications, discovering bottlenecks. In other words, traces tell DevOps teams where issues are occurring in their environments. They’re the foundation of proactive monitoring. By analyzing traces, engineers can discover which metrics or logs are related to a particular issue, mitigating future issues.
For example, traces that help identify slow processes include API queries, front-end API traffic, server-to-server workloads, and internal API calls.
While metrics, logs, and traces offer users valuable application and system performance data, these signals don’t always provide the details required for troubleshooting code and performance tuning. This is where profiles come in.
Profiles
Profiling is the gathering and analysis of profiles — stack traces that help identify issues related to data structures, code visibility, and memory allocation at the kernel and user levels.
Profiling helps uncover bottlenecks across your system at the code level, another key benefit of modern observability. OpenTelemetry is also adopting profiling as a signal. As a result, profiling is emerging as the fourth and newest pillar of observability.
Essential observability metrics: The 4 golden signals for SRE teams
While every organization’s monitoring needs are unique, certain observability metrics are universally important. These metrics are sometimes referred to as the four golden signals within the site reliability engineering (SRE) community.
Latency
Latency measures the time it takes for data to travel from one point to another. Latency will signal underlying performance issues. High latency can degrade user experiences by increasing load times, causing application errors, and challenging user expectations.
Traffic
Traffic metrics track the volume of requests or transactions an application processes. They help teams understand user behavior and anticipate scaling needs.
Errors
Error metrics provide visibility into failed requests or operations. Monitoring error rates and identifying patterns can help address recurring issues.
Saturation
Saturation metrics indicate how close a system is to its capacity limits. Monitoring resource utilization ensures that engineers can proactively address bottlenecks before they impact performance.
These four golden signals are key to effective observability practices as they provide insights into the health and performance of IT systems. When monitored, correlated, and analyzed, these metrics help IT teams get actionable insights that enable them to take on a more proactive stance around site reliability and performance monitoring.
Best practices for implementing observability metrics
The primary challenge of implementing observability metrics has to do with sorting through the noise — many signals produce a mass of telemetry data that may not all be useful. In addition to this, SREs will often struggle with data heterogeneity. How do you correlate various types of disparate data for easier troubleshooting?
From these challenges, we can establish some best practices for implementing observability metrics.
Define clear objectives: Successfully implementing observability metrics — and combatting data overwhelm — begins with establishing your goals. To define these objectives, ask yourself what you need your metrics to tell you. You don’t need to monitor everything; you only need to monitor what is important to your organization and systems.
Use open standards to instrument your applications: Instrumentation is the process of generating and collecting telemetry data from applications. To avoid vendor lock-in when you instrument your applications, consider a vendor-neutral framework like OpenTelemetry (OTel). OTel provides a standardized framework that enables you to collect and compare telemetry data from multiple sources.
Leverage automation: Automate data collection, analysis, and alerting to reduce manual effort and enable faster response times.
Customize visualizations: In order to meet your defined objectives, it’s best to customize your dashboards. Default dashboards are only useful to a point — customizing how you visualize your environment is key to successful observability.
Observability metrics with Elastic
Elastic Observability provides a unified solution for collecting, monitoring, and analyzing observability metrics across your technology stack. With Elastic Observability, you can collect, store, and visualize observability metrics from any source and speed up problem resolution with our Search AI Platform.
Elastic Observability prevents outages and accelerates problem resolution with search-based relevance, no-compromise data retention, improved operational efficiency and cost, and a future-proofed investment. Get fast, contextual, and unified insights across the broadest data sources with an open, OTel-first solution that seamlessly integrates with your evolving technology ecosystem.
Learn more about observability with Elastic.
Deep dive into more observability metrics resources
The release and timing of any features or functionality described in this post remain at Elastic's sole discretion. Any features or functionality not currently available may not be delivered on time or at all.