Generative AI hype: Debunking 4 myths for IT leaders
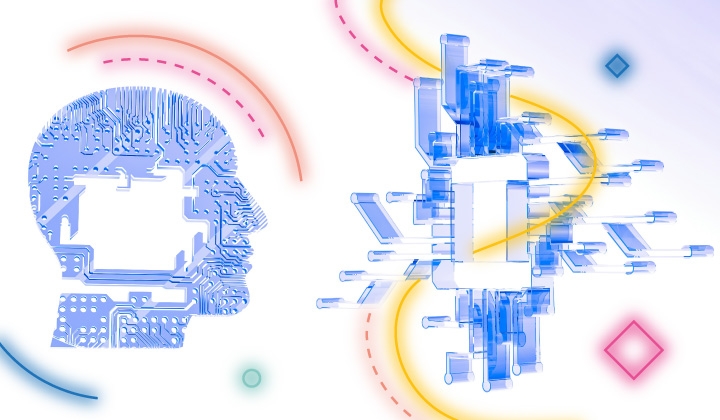
There’s no denying it: generative AI (GenAI) is reshaping the future of IT (and possibly the world). According to a 2024 pulse survey from Forrester, 67% of AI decision-makers say their organization plans to increase investment in generative AI in the coming year.1 So what’s just generative AI hype and what’s worthy of long-term investment? And how can IT and business leaders separate fact from fiction?
The buzz brings both excitement and skepticism. There are questions about how generative AI collects data, its safety measures, and its impact on IT and business operations. Let’s explore the real capabilities of generative AI, debunk common myths, and show how you can responsibly harness its potential beyond the generative AI hype.
Is generative AI overhyped?
It depends on how you want to use it. And the data the generative AI model is using. Some use cases don’t call for generative AI but rather traditional AI. For example, if your organization goes through quality control checks for products shipped out, you might consider a traditional AI-enabled rule-based system instead of generative AI, as checking products against a set of standards is a straightforward process. In these simpler use cases, generative AI’s ability to improve quality control efficiencies is overhyped.
On the other hand, if generative AI can be used to expand the wealth of knowledge to help someone make better decisions, then it might be worth the investment. Imagine your organization enriching a large language model (LLM) with your proprietary data, like your internal company wiki, HR policies, and corporate presentations. Generative AI can help employees self-serve by asking questions in natural language. This practical use case can enable your teams to recoup time finding information for more strategic tasks and complex problems. It’s already been successful at Elastic — our internal generative AI assistant, ElasticGPT, has saved employees more than five hours per month or 63 hours annually.
As for the future of generative AI hype, the Gartner hype cycle predicts that by 2026, more than 80% of enterprises will have used generative AI APIs or models and/or deployed GenAI-enabled applications in production environments.2 That’s up from less than 5% in 2023. As generative AI use cases continue to expand, you’ll need to see through the hype and focus on use cases that will have the most impact on your business.
Let’s jump into what’s generative AI myth and what’s reality so you can create and refine a strategy that ignores the hype and focuses on long-term success.
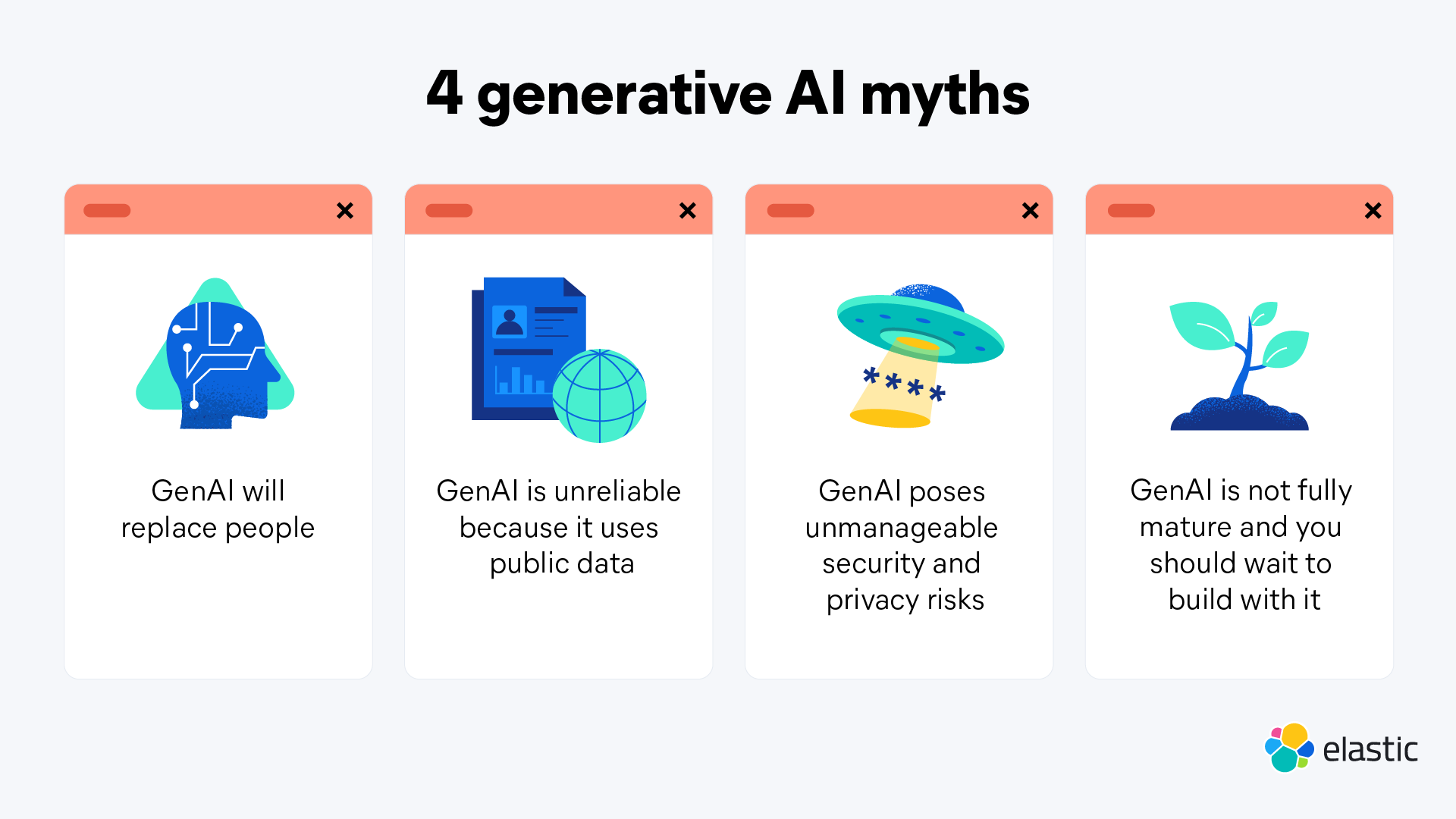
Myth: Generative AI will replace people
Reality: AI assistants can provide actionable insights to employees.
You won’t hear about generative AI hype without hearing of its potential to replace employees. Generative AI technologies augment your team’s expertise and workflows — they don’t replace them. While a generative AI-powered assistant offers insights, automates repetitive tasks, and improves response times, human intuition and problem-solving capabilities remain critical. IT analysts, security teams, and customer support representatives still provide the context, judgment, and nuanced understanding of your organization’s systems, the threat landscape, and business priorities that only human expertise can bring.
AI assistants can focus on repetitive tasks to free up employees to take on more challenging projects. McKinsey estimates $4.4 trillion in added productivity growth potential3 from corporate use cases. A site reliability engineer (SRE) might use an AI assistant to investigate your network issues to understand the root cause. This AI-assisted workflow can help SRE’s quickly resolve downtime impacts and improve system reliability. Now, the SRE can focus on resolving issues or reclaiming time back to focus on complex IT projects that require human ingenuity.
Myth: Generative AI is unreliable since it uses public data
Reality: You can enhance your generative AI models with your own proprietary data through retrieval augmented generation (RAG).
While public internet data powers many AI applications, generative AI models can securely use your organization’s proprietary data to provide tailored responses and a variety of use cases. This approach — using RAG — combines internal, vetted data with generative capabilities to deliver results that are highly relevant and actionable. This lessens the probability of hallucinations and ensures that responses are unique to your organization, not based on whatever exists on the web.
In security operations, where teams face hundreds or even thousands of alerts daily, generative AI tools can help cut through the noise. With a generative AI-powered assistant that is enhanced with proprietary data, security analysts only have to investigate filtered alerts by receiving intelligent summaries and access to guided next-best actions that streamline response times. The assistant can go further by keeping context after each query, allowing analysts to build insights iteratively and add findings to a case with ease. This data is then fed back into the model through RAG, continuously improving the system and ensuring analysts are working with the most accurate information.
Myth: Generative AI poses unmanageable security and privacy risks
Reality: Regulated generative AI systems are designed with robust security measures, such as encryption, access controls, and compliance with data protection regulations.
While security and privacy concerns are valid, they are not insurmountable. Generative AI systems should and can be designed with robust security measures. Using techniques like RAG, generative AI can source information from your proprietary data securely using access controls. This gives you control over data privacy and ensures your AI outputs are reliable, role-based, and context-specific to your organization. IT leaders can also implement private AI models that operate within their own infrastructure and encrypt PII information to minimize risks.
As sensitive information is potentially collected, stored, and used to train machine learning models, multiple compliance laws are in place to protect users’ data. The European Union’s General Data Protection Regulation (GDPR), the EU Artificial Intelligence (AI) Act, US regulations, and China’s Interim Measures for the Administration of Generative AI Services should be incorporated into a compliance strategy.
As the technology evolves, so will the laws. So it’s important to always be on top of AI compliance and risks. Bill Wright, Elastic’s senior director of global government affairs, says, “Initiatives, such as regulatory sandboxes, independent algorithm audits, and the adoption of responsible design principles, can help create an environment where AI is developed and deployed safely.”
Myth: You should wait until generative AI is more mature before you use or build with it
Reality: There are safe and secure ways to implement generative AI into your systems today.
In fact, your competitors are already using it. A recent study found that 93% of C-suite executives have already deployed or are planning to invest in generative AI. These leaders also believe they will increase annual revenue when they can ingest data in real time, use data analytics tools for business decision-making, and use AI for data-driven insights.
McKinsey found that two-thirds of IT leaders launched their first generative AI initiative over a year ago.3 Erik Brynjolfsson, professor at Stanford University and director of the Digital Economy Lab at the Stanford Institute for Human-Centered Artificial Intelligence (HAI), says, “Now is the time to be getting benefits [from AI] and hope that your competitors are just playing around.”
Here are some examples of organizations using and succeeding with generative AI today:
EY built a generative AI experience that helps clients unlock insights from unstructured data. EY is able to help banks streamline reporting on their ESG commitments by extracting key insights from documents across the organization.
IBM is using generative AI to give businesses the tools to combine proprietary documentation with LLMs. This ensures outputs are enriched with business context, making them more relevant and helpful to the user.
- Stack Overflow uses generative AI to power its OverflowAI tool, which draws on data held in the public platform and a company's private instance of Stack Overflow.
Generative AI hype will continue
GenAI isn’t going anywhere. The hype around it may die down as new technologies take the spotlight. Set yourself up for success now by laying a solid foundation for your data. It’s crucial for you as an IT and business leader to separate fact from fiction to harness its potential responsibly and future-proof your business. Generative AI is a powerful tool that, when implemented thoughtfully, can enhance productivity, improve decision-making, and drive innovation — all while maintaining robust security and compliance standards.
By focusing on long-term strategies that prioritize scalability, data privacy, and ethical use, you can confidently integrate generative AI into your organization. The key lies in building a solid foundation, staying informed, and continuously adapting to the evolving landscape. Generative AI is not just a fleeting trend; it’s a transformative force that, when approached with care and foresight, can deliver lasting value.
Ready to see what is possible with generative AI? Check out our two-question assessment to see how generative AI can drive change at your organization.
Additional resources
Sources
Forrester, Generative AI Trends For Business: Why, When, And Where To Begin.
Gartner, What’s Driving the Hype Cycle for Generative AI, 2024.
McKinsey, Superagency in the workplace: Empowering people to unlock AI’s full potential, 2025.
The release and timing of any features or functionality described in this post remain at Elastic's sole discretion. Any features or functionality not currently available may not be delivered on time or at all.
In this blog post, we may have used or referred to third party generative AI tools, which are owned and operated by their respective owners. Elastic does not have any control over the third party tools and we have no responsibility or liability for their content, operation or use, nor for any loss or damage that may arise from your use of such tools. Please exercise caution when using AI tools with personal, sensitive or confidential information. Any data you submit may be used for AI training or other purposes. There is no guarantee that information you provide will be kept secure or confidential. You should familiarize yourself with the privacy practices and terms of use of any generative AI tools prior to use.
Elastic, Elasticsearch, ESRE, Elasticsearch Relevance Engine and associated marks are trademarks, logos or registered trademarks of Elasticsearch N.V. in the United States and other countries. All other company and product names are trademarks, logos or registered trademarks of their respective owners.