Detecting privileged access activity: A new Kibana integration
The new advanced detection analytics package to detect privileged access activity
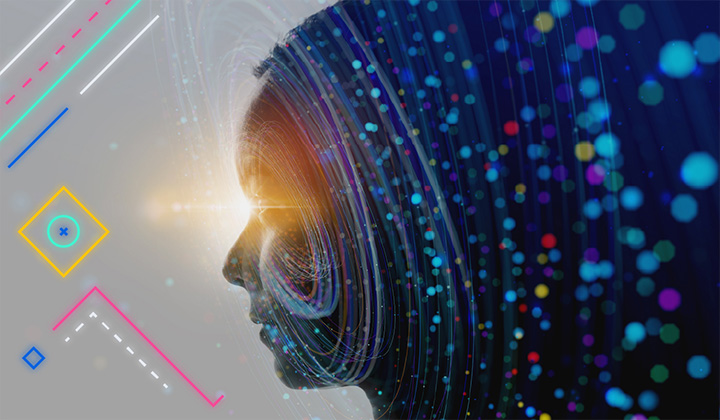
Privileged accounts are a prime target for attackers, often exploited to accelerate access and move laterally within networks. Given the prevalence of access brokers who satisfy a nearly endless demand for stolen credentials, organizations need robust detection mechanisms to identify suspicious privileged activities as early in an intrusion attempt as possible.
This blog post introduces the newly released Privileged Access Detection package, now available as a technical preview under Kibana's beta integrations in version 8.18 and 9.0. This integration includes ingest pipelines, transforms, and ML jobs designed to detect anomalous privileged activities across Windows, Linux, and Okta environments. Additionally, dedicated dashboards highlight key details such as entities, event codes, process names, IP addresses, and geolocations, providing an intuitive view of detected anomalies. These capabilities empower security teams to efficiently investigate and identify suspicious privileged access and administrative actions.
Introduction to Privileged Access Detection
Privileged access activity includes actions performed by users with elevated permissions, such as system administrators or service accounts. These users can modify system configurations, access sensitive data, manage user roles, install software, change security policies, and directly interact with critical controls.
Traditional security measures rely on predefined rules and signatures, which can fail to detect new or sophisticated attack patterns. Since privileged users perform a wide range of administrative tasks, distinguishing between legitimate and suspicious activities can be challenging. The Privileged Access Detection package uses machine learning to establish baseline behaviors for users and entities, allowing it to detect anomalies that deviate from typical usage patterns. Currently, it focuses on detecting anomalous privileged access activities from valid accounts, such as admin-based events and commands.
Our approach
At Elastic, we focus on detecting abnormal behaviors exhibited by valid privileged accounts that have elevated access and control over critical systems. Instead of simply monitoring isolated actions, we track privileged account activities in the context of administrative behaviors. By analyzing typical access patterns, we can flag activities that deviate from the norm, such as unusual access from different IPs, devices, or locations. We also pay close attention to multiple concurrent sessions from the same account, as these could indicate unauthorized use or suspicious activity.
The workflow of this package, as shown in the image below, outlines the key processes: data preparation, featurization, and machine learning. In the following sections, we’ll dive deeper into each phase of the workflow.
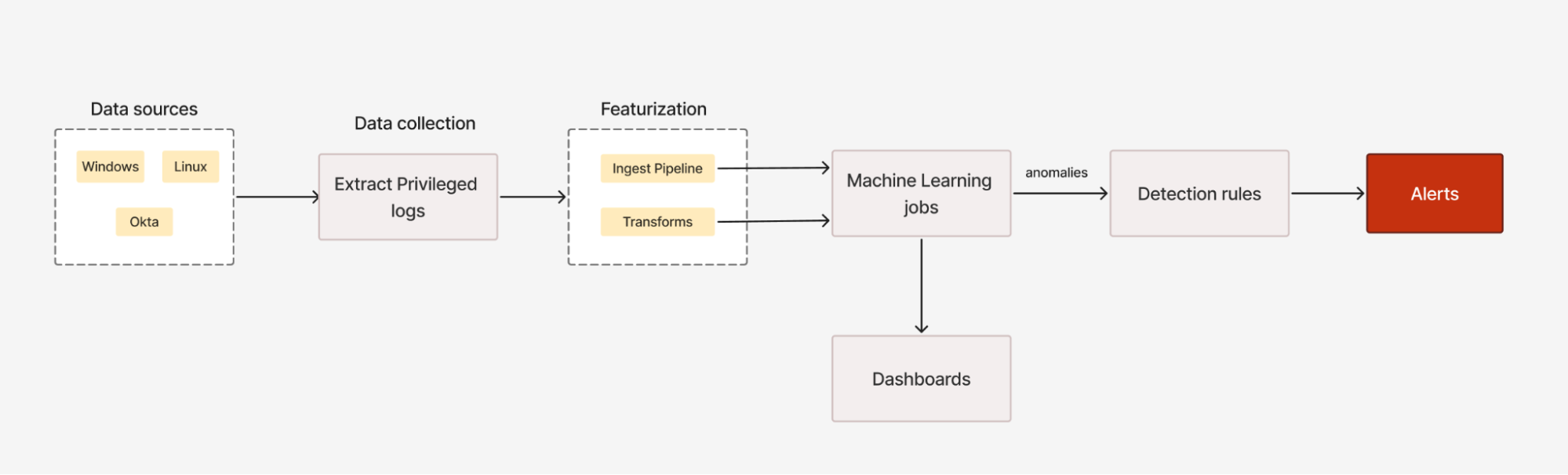
Data preparation
This package is designed for hybrid IT environments, covering Windows, Linux, and Okta systems. To effectively monitor privileged activities across these diverse platforms, we extract relevant event types and commands, aligning with MITRE ATT&CK techniques for Privilege Escalation. The below table demonstrates how different data sources correspond with particular privileged event types and MITRE ATT&CK techniques.
Data Source | Privileged Event Types | MITRE ATT&CK Coverage |
Windows | Account Management Events, Group and Role Management Events, Special Logon Events | T1078 (Valid Accounts), T1068 (Exploitation for Privilege Escalation), T1098 (Account Manipulation) |
Linux | Privileged commands executed by admin accounts | T1078 (Valid Accounts), T1068 (Exploitation for Privilege Escalation), T1098 (Account Manipulation) |
Okta | User Lifecycle Change Events, Group Membership Change Events, Group Privilege Change Events, User Account Change Events, Group Lifecycle Change Events | T1078 (Valid Accounts), T1068 (Exploitation for Privilege Escalation), T1098 (Account Manipulation), T1069 (Permission Groups Discovery) |
Featurization
The next step after data preparation is featurization, where raw privileged event logs are transformed into usable features. Features are created using Elasticsearch ingest pipelines and pivot transforms and are then analyzed by machine learning models. For example, we calculate the Shannon Entropy of the process.command_line field. This metric evaluates the randomness of command text and identifies suspicious behavior when a user's entropy score is significantly higher than usual, which indicates that their commands are unusually complex and potentially anomalous.
The below painless script computes the entropy for Linux command lines and stores the result under the process.command_line_entropy field.
String inputString = ctx["process"]["command_line"];
Map m = new HashMap();
for (int i = 0; i < inputString.length(); i++) {
char c = inputString.charAt(i);
m.put(c, m.getOrDefault(c, 0) + 1);
}
double result = 0.0;
for (entry in m.entrySet()) {
double frequency = entry.getValue() / (double) inputString.length();
result -= frequency * (Math.log(frequency) / Math.log(2));
}
ctx["process.command_line_entropy"] = result;
Additionally, we employ two pivot transforms: one for Windows and one for Okta. For Windows, the transform focuses on the winlog.event_data.PrivilegeList field, filtering the most significant privileges assigned to a user. We then monitor for spikes in the assigned privileges or for rare privileges being granted to a user, which could signal anomalous behavior. The Okta transform identifies multiple concurrent sessions initiated by the same user from different IPs and locations, feeding this data into the machine learning models to learn the user's typical session patterns.
Machine learning
Finally, the features created during the data preparation and featurization steps are used in anomaly detection jobs to uncover unusual patterns in user behavior. This package includes a total of 21 anomaly detection jobs, with 9 each targeting Windows and Okta and 3 for Linux. These jobs are designed to detect a variety of suspicious activities, such as spikes in group and user management changes, unusual privilege grants, rare source IPs and regions accessed by privileged users, and uncommon privileged process names executed. For a more detailed overview of these jobs, check out the README link for the complete catalog of anomaly detection jobs with their descriptions.
Getting started
As of 8.18 (and 9.0), the Privileged Access Detection package is available as a beta-release under the Integrations app in Kibana. To set up and start using this package, follow the steps below.
Step 1: Installing the package
You will be able to view the package after enabling the Display beta integrations option at the bottom of the Integrations app.
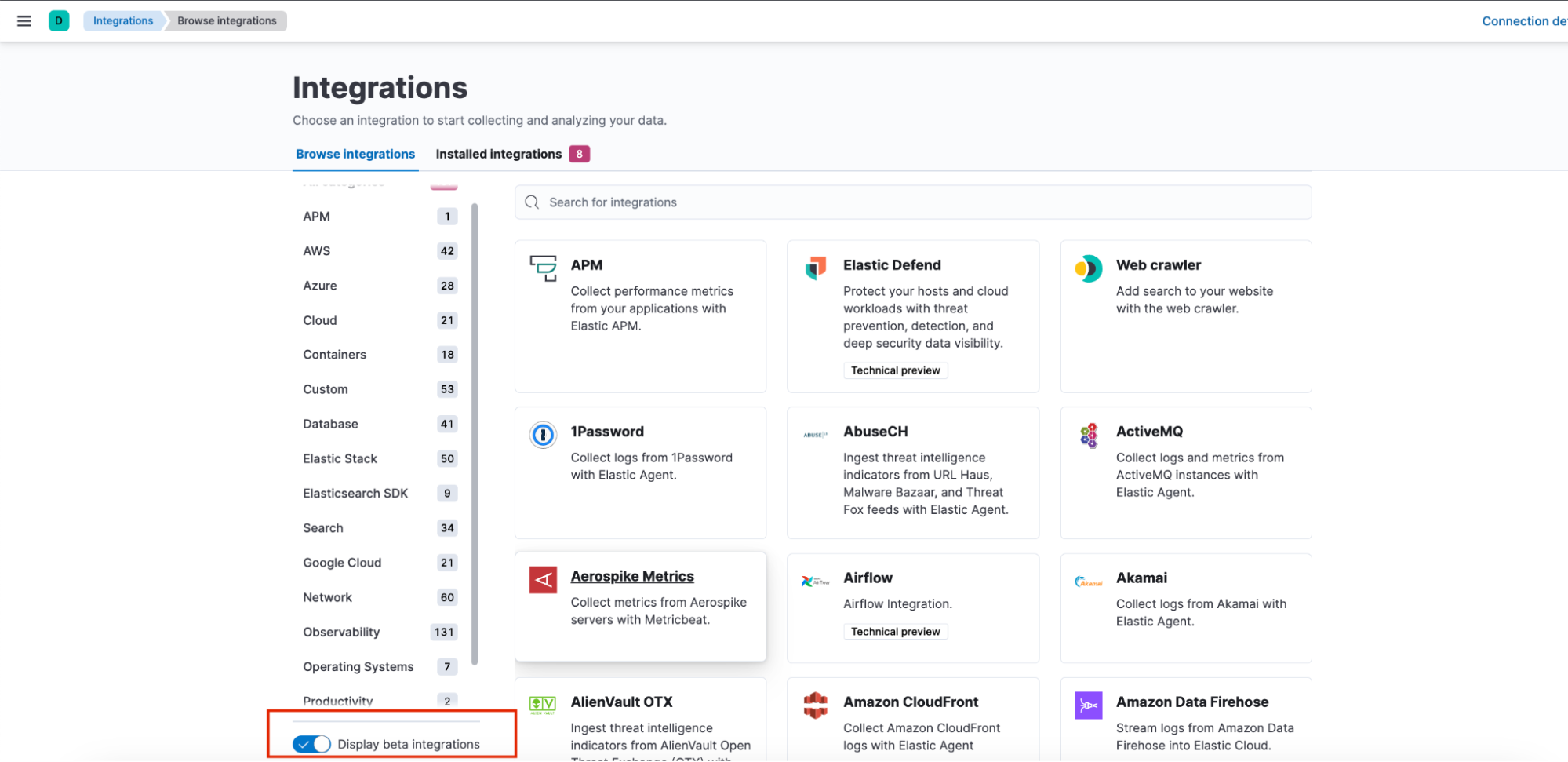
After this option is enabled, search for Privileged Access Detection. To install, go to the Settings tab and click on Install Privileged Access Detection assets. This will install all the artifacts under this package.
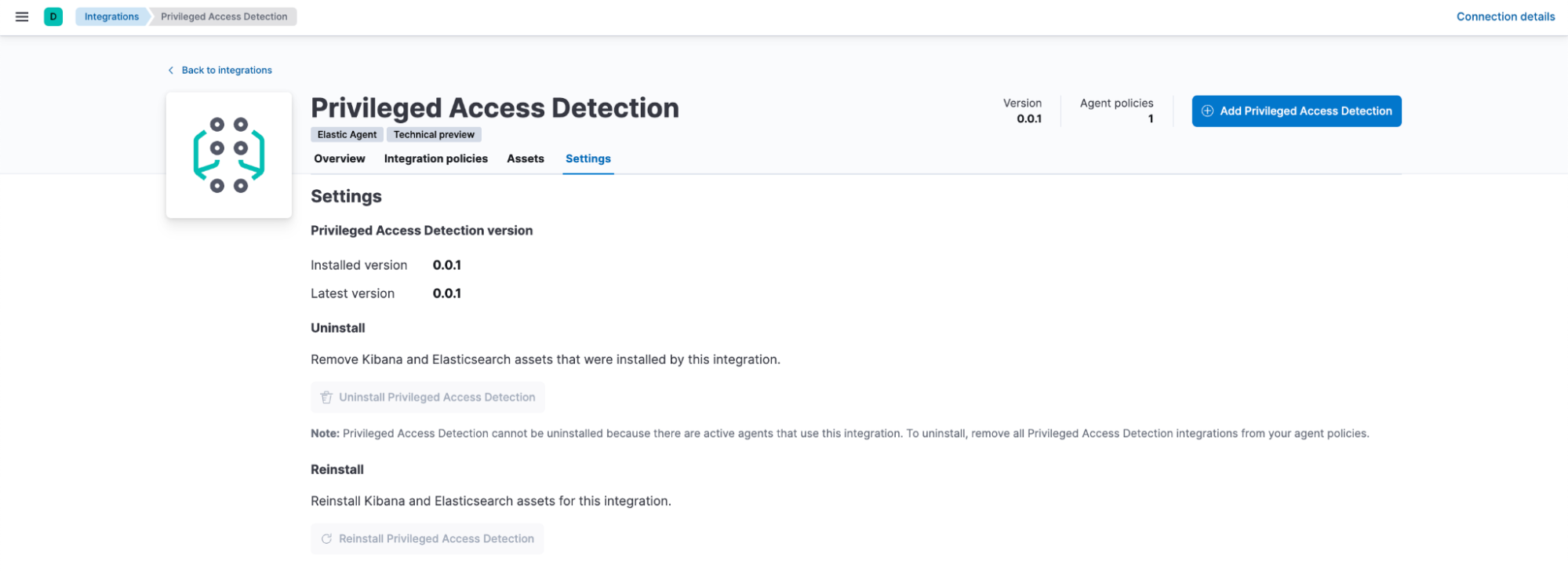
Step 2: Configuring transforms and ingest pipeline
Once the installation is complete, you can find the 0.0.1-ml_pad_ingest_pipeline ingest pipeline by navigating to Stack Management > Ingest Pipelines. The pivot transforms can be located under Stack Management > Data > Transforms. To begin collecting data using the ingest pipeline, follow the instructions located in steps 2, 3, and 4 of the installation section in the package’s overview. For instructions related to the transform, refer to the Transform section of the overview.
Step 3: Starting anomaly detection jobs
The anomaly detection jobs under this package are only visible if your cluster is actively ingesting Windows, Linux, or Okta logs that match the KQL query below.
((host.os.type: "windows" or host.os.type: "Windows") and exists(winlog.event_id)) or
((host.os.type: "linux" or host.os.type: "Linux") and event.category: "process") or data_stream.dataset: "okta.system" or exists(okta_distinct_ips) or exists(privilege_type)
Ensure that Step 2 is complete and the transforms are set up correctly before creating Data Views for anomaly detection jobs. To create the Data Views, follow the steps located in step 6 of the installation section in the package's overview.
After creating the Data View for anomaly detection jobs, navigate to Machine Learning > Anomaly Detection and select the Jobs wizard. Click on Create job and choose the Data View you created. The Privileged Access Detection card will be visible under the Use preconfigured jobs option.
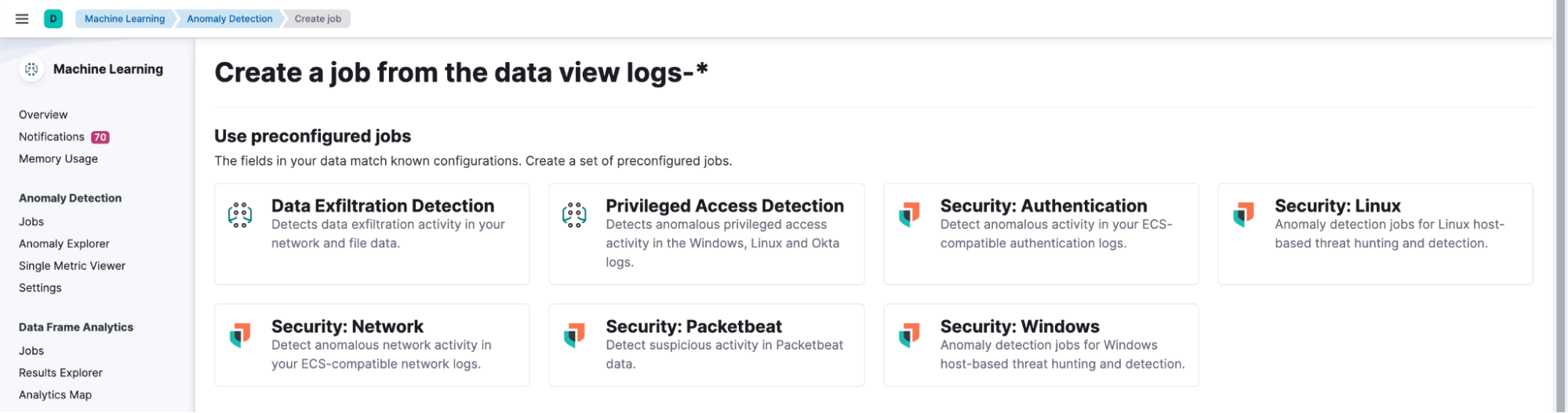
Select the card and click on the Create jobs button.
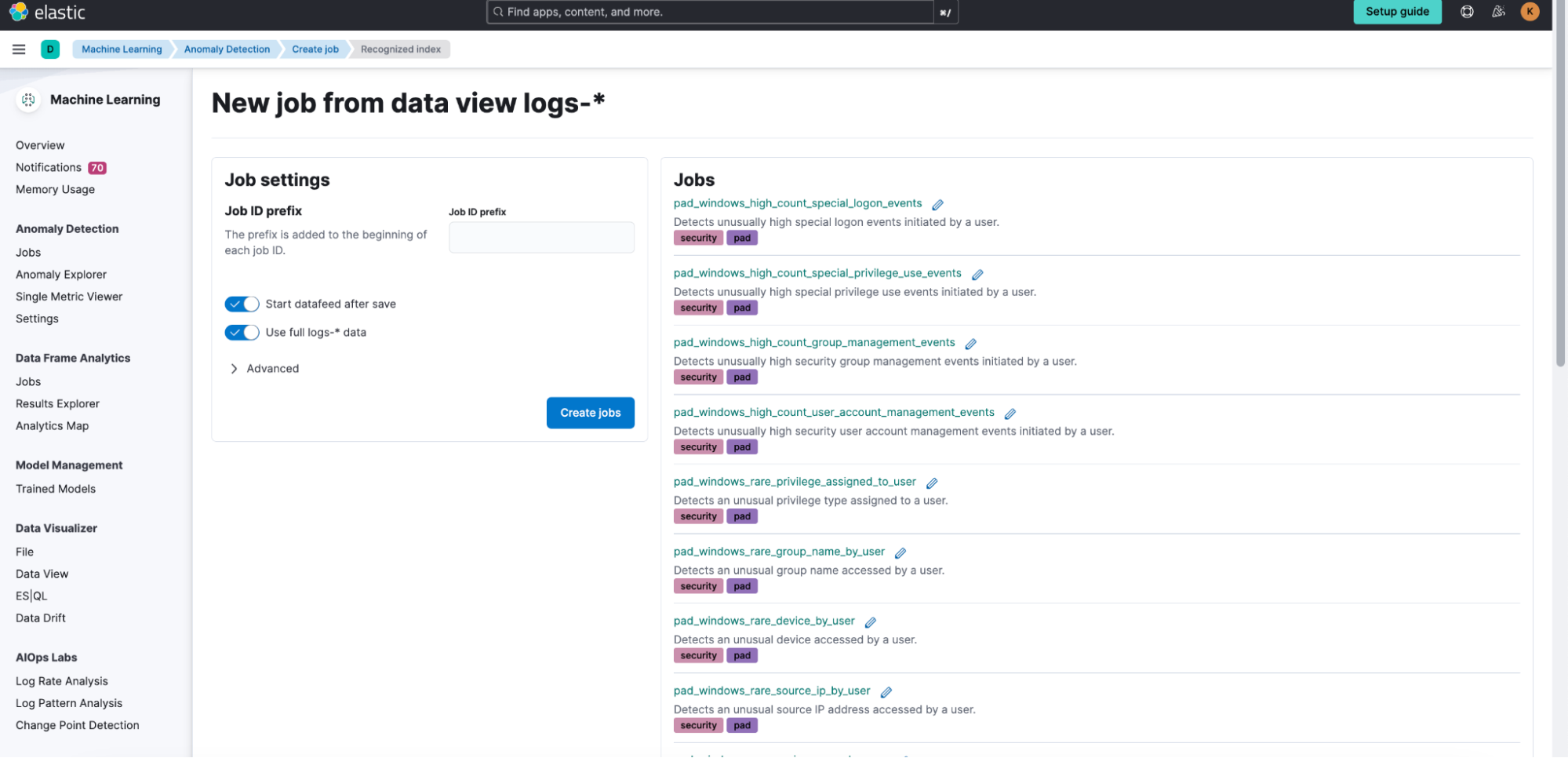
Step 4: Enabling Security Detection rules
Ensure that the machine learning jobs from the previous step are running. Then, navigate to Security > Rules > Detection rules (SIEM) to enable the 21 detection rules associated with the Privileged Access Detection package.
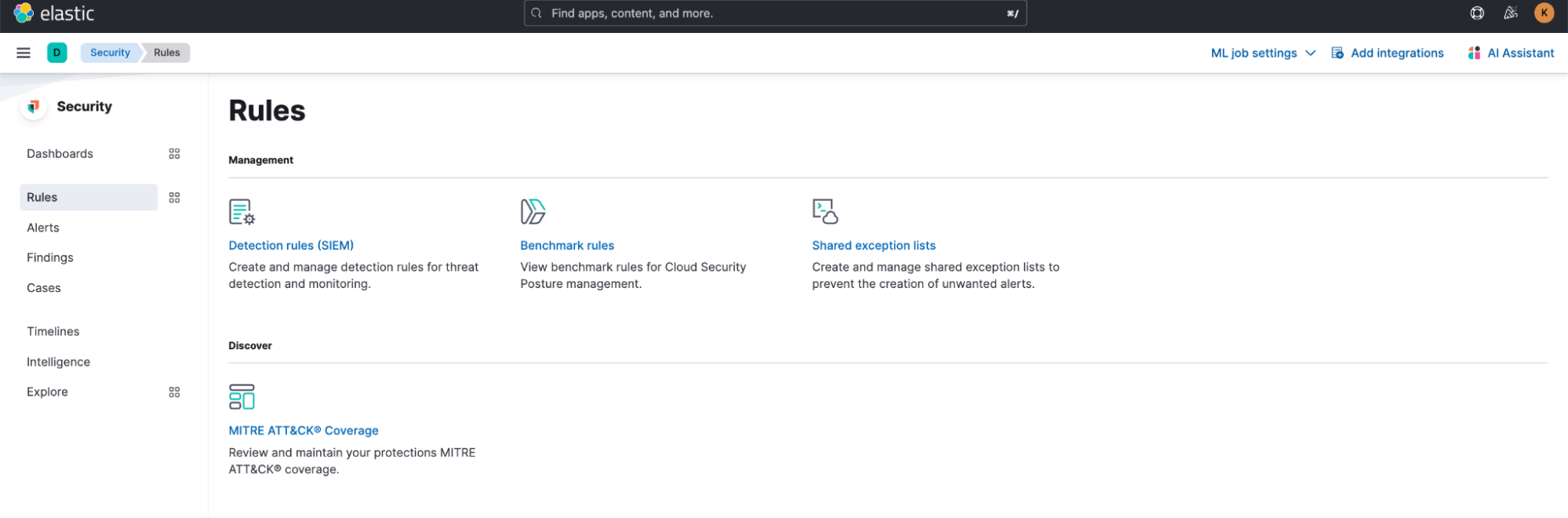
Use the Privileged Access Detection tag filter to find the relevant rules within this package. Select the rules that you want to activate, and then choose Enable from the Bulk actions menu.
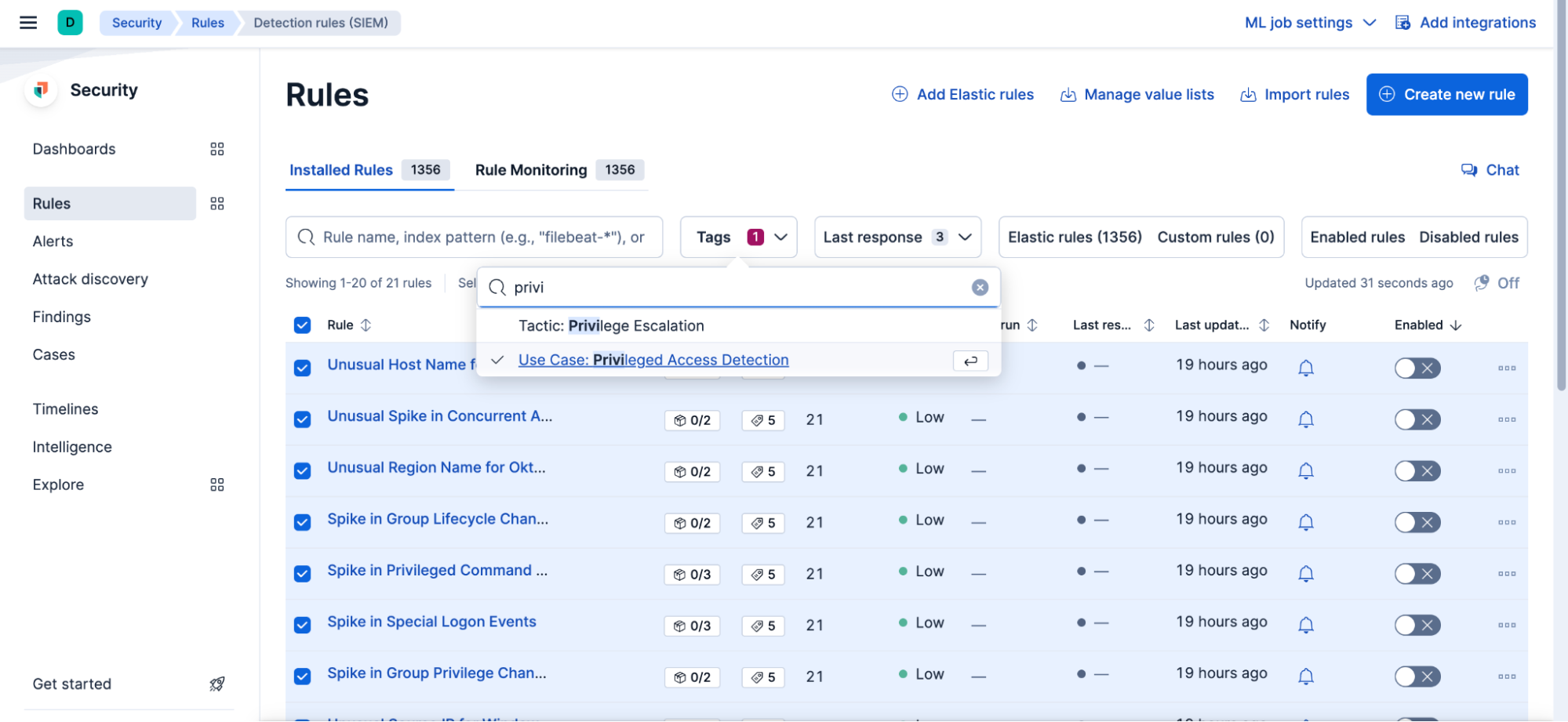
Start analyzing the anomaly detection alerts
You can track the anomalies triggered by the jobs under Anomaly Detection > Anomaly Explorer.
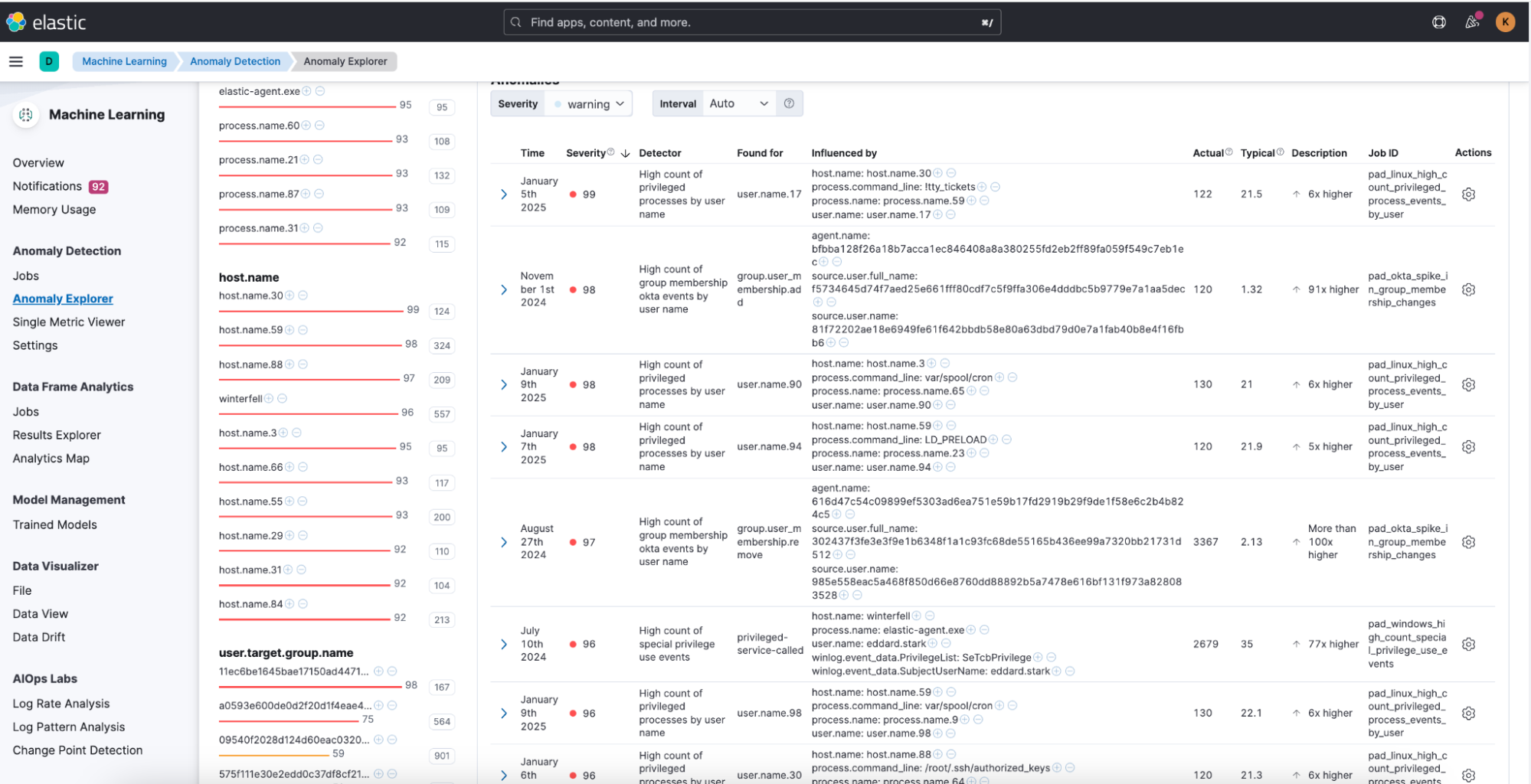
For a more comprehensive view of the generated anomalies and a complete contextual understanding of the alerts, utilize the dashboards included in this package. You can access the Privileged Access Detection dashboards under Analytics > Dashboards to gain a 360-degree perspective on the detected anomalies.
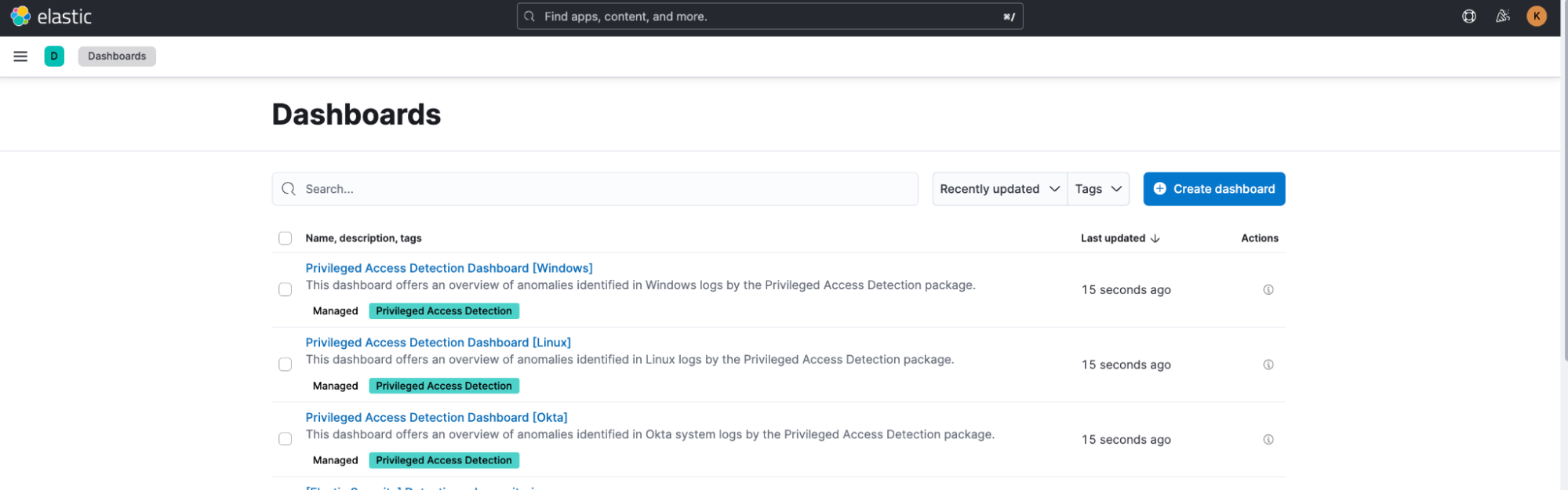
To configure and begin using the dashboards, adhere to the instructions outlined in step 8 of the installation section on the package's overview.
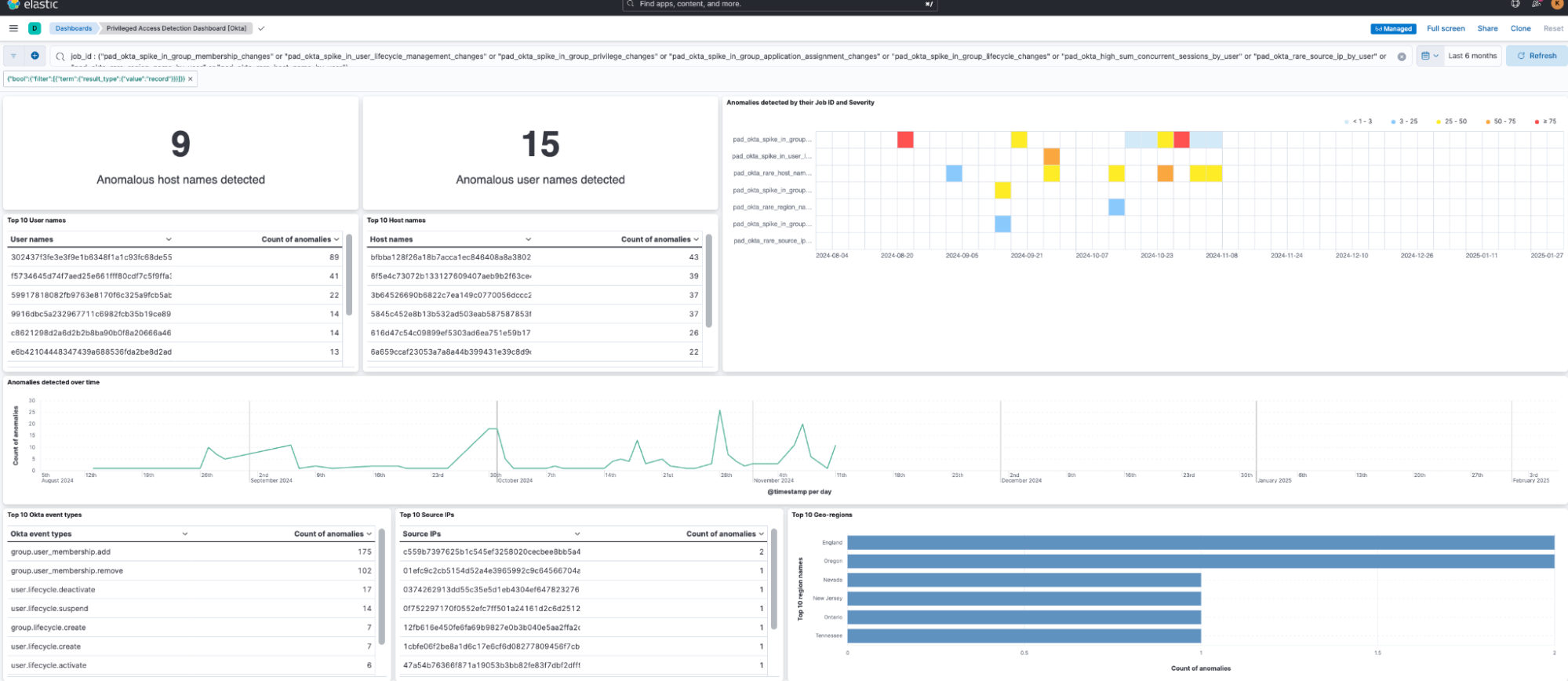
Wrapping up
In this blog post, we introduced our new Privileged Access Detection capability, which uses Elasticsearch components to identify unusual privileged activity in complex IT environments. We explained how these components fit within the package's architecture, and provided installation instructions and key steps to help you begin analyzing and addressing anomalies detected by the machine learning jobs.
We'd love for you to try out the Privileged Access Detection package and give us feedback as we work on adding new capabilities to it. If you run into any issues during the process, please reach out to us on our community Slack channel or discussion forums.
The release and timing of any features or functionality described in this post remain at Elastic's sole discretion. Any features or functionality not currently available may not be delivered on time or at all.