Powering the future of financial services with AI-driven intelligence
Learn how financial institutions can harness RAG, vector search, and Elastic’s Better Binary Quantization to drive AI efficiency, reduce costs, and deliver real-time, accurate insights at scale.
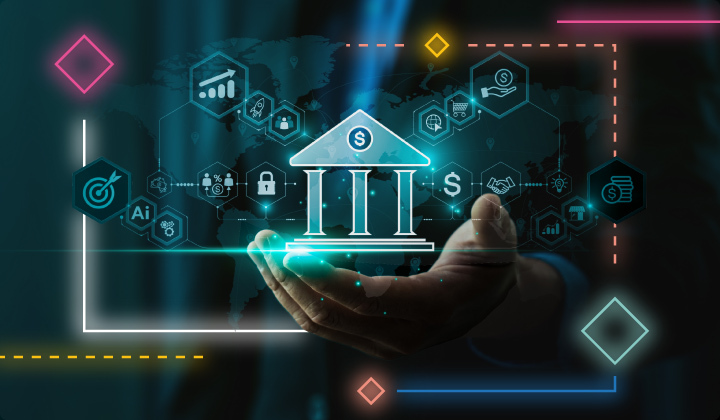
Get your data foundation right: The key to AI success in financial services
You know the old saying, “Garbage in, garbage out.” No matter which top tech company is discussing the future of AI in financial services, one consistent theme emerges — if your data foundation isn’t solid, your AI initiatives will fail.
There’s also been plenty of talk lately about CFOs and CIOs/CTOs clashing over AI spending. When it comes to AI investments, companies want results — and they want them fast. CFOs aren’t likely to sign off on AI funding if it doesn’t show measurable ROI within a pretty aggressive timeframe.
If IT leaders want CFOs to back AI investments, they must demonstrate clear ROI, whether in cost savings or efficiency gains. The only way for financial services firms to launch successful AI projects is to harness their vast data assets effectively — and that requires the right tools to structure, retrieve, and analyze data for meaningful AI-driven outcomes.
It’s all about data
The ability to ingest massive amounts of internal and external data is a basic need in financial services. As J.P. Morgan CEO Jamie Dimon recently said in a speech at the Stanford Graduate School of Business, discussing J.P. Morgan’s AI strategy with regard to the value of data, “We have huge data, a lot of the world’s data is private … so we can run ML and AI against our [private] data and outside data … and I only want to use it to help my customer.”
A vector database is essential for AI-driven financial services because of its unique ability to store and synthesize massive amounts of structured and unstructured data — grouped by similarity using machine learning techniques. Elastic, the most downloaded vector database in the world, is widely known among developers for its ability to organize financial data semantically, making it the ideal foundation for AI-powered insights.
Retrieval augmented generation leads the way
This is where retrieval augmented generation (RAG) comes in. RAG acts as the connective tissue between internal data and large language models (LLMs), dynamically retrieving the most relevant data to enhance AI’s accuracy and relevance. In financial services, RAG is a game-changer:
Improves decision-making: Provides AI models with real-time, contextually relevant information for better risk assessments, fraud detection, and compliance monitoring
Bridges structured and unstructured data: Synthesizes financial data across multiple formats (transaction records, regulatory filings, emails) for a holistic analytical view
Enhances customer interactions: Powers AI-driven financial recommendations, faster query resolution, and personalized insights by pulling relevant data from knowledge bases and transaction histories
Ensures compliance and transparency: Helps track and validate data sources, improving auditability and regulatory compliance for AI-driven decisions
- Boosts AI model performance: Continuously enriches AI models with real-time data, reduces hallucinations, and improves accuracy in applications like automated underwriting, risk scoring, and anomaly detection.
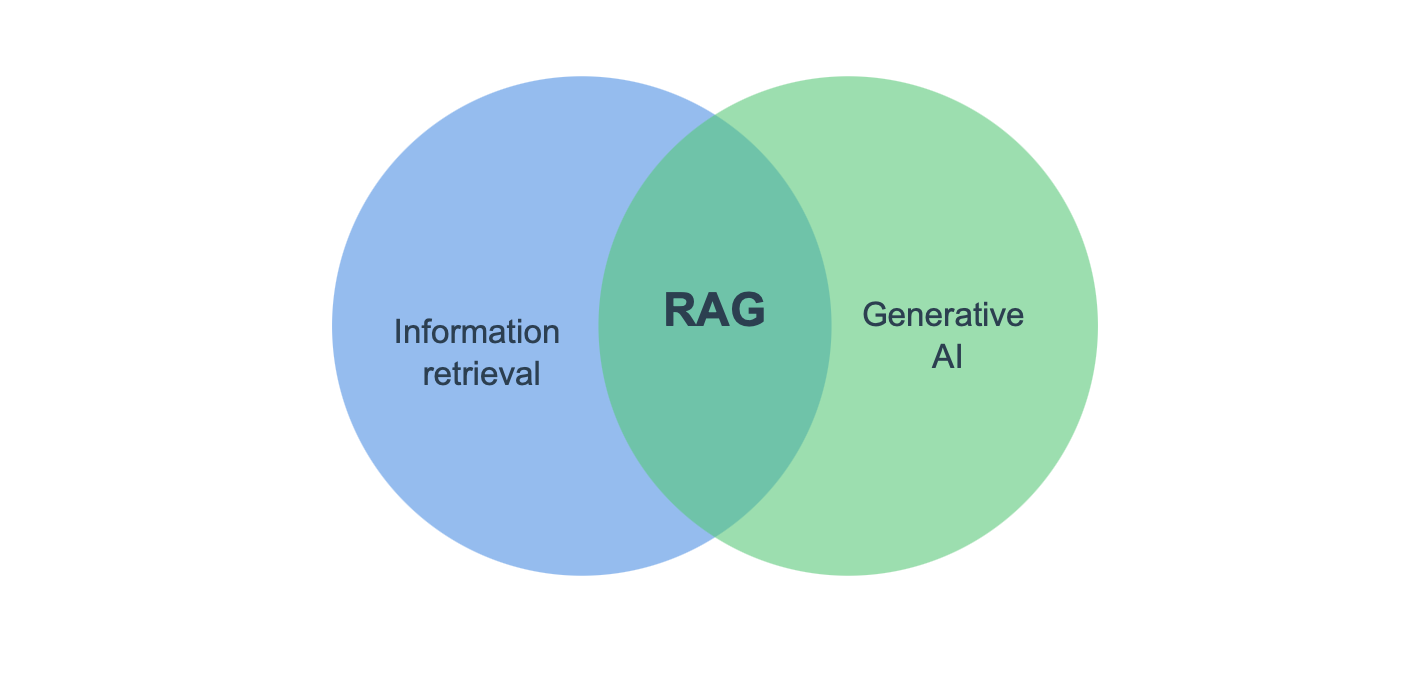
Introducing BBQ: Better Binary Quantization for AI-driven efficiency
While RAG enables more precise AI responses, financial services companies face another challenge — scalability. AI-driven processes require vast amounts of memory, making large-scale vector search expensive. That’s why Elasticsearch developed BBQ (Better Binary Quantization), a breakthrough in vector compression.
BBQ compresses vectors by 32x, delivering an astonishing 95% reduction in memory usage while maintaining lightning-fast search speeds and no loss in recall. This efficiency translates into lower costs and faster AI-driven insights — a critical advantage in financial services.
Since its debut in Elasticsearch 8.16, BBQ has evolved with an updated algorithm, now delivering up to 20% recall gains in certain datasets. In head-to-head performance tests against OpenSearch FAISS, OpenSearch’s binary quantization method, Elasticsearch BBQ is 5x faster and delivers 3.9x greater throughput across all recall levels — without compromising accuracy.
Operationalizing data for AI success in financial services
By integrating vector search, RAG, and advanced quantization techniques like BBQ, financial institutions can unlock the full potential of AI — delivering smarter insights, optimizing costs, and ensuring compliance in an ever-evolving regulatory landscape.
AI success starts with a strong data foundation — and Elastic provides the tools to make it happen. Ready to see the impact of smarter AI in financial services? Visit elastic.co/industries/financial-services or contact us at https://www.elastic.co/industries/financial-services/contact to learn more.
Watch: Elastic's Generative AI Momentum
Learn more about building RAG systems using Elasticsearch:
- Beyond RAG Basics: Advanced strategies for AI applications
- Building a RAG system with Gemma, Hugging Face, and Elasticsearch
- Building an agentic RAG tool with Elasticsearch and Langchain
The release and timing of any features or functionality described in this post remain at Elastic's sole discretion. Any features or functionality not currently available may not be delivered on time or at all.
In this blog post, we may have used or referred to third party generative AI tools, which are owned and operated by their respective owners. Elastic does not have any control over the third party tools and we have no responsibility or liability for their content, operation or use, nor for any loss or damage that may arise from your use of such tools. Please exercise caution when using AI tools with personal, sensitive or confidential information. Any data you submit may be used for AI training or other purposes. There is no guarantee that information you provide will be kept secure or confidential. You should familiarize yourself with the privacy practices and terms of use of any generative AI tools prior to use.
Elastic, Elasticsearch, ESRE, Elasticsearch Relevance Engine and associated marks are trademarks, logos or registered trademarks of Elasticsearch N.V. in the United States and other countries. All other company and product names are trademarks, logos or registered trademarks of their respective owners.